Handbook of The Vehicle Routing Problem
The University of Bologna’s Faculty of Engineering is home to Professor of Combinatorial Optimization Paolo Toth. His current areas of interest include the development of algorithms for combinatorial optimization and graph theory issues as well as their use in actual transportation, crew management, routing, and loading issues. The Euro Gold Medal honor was bestowed upon him in July 1998. In addition to co-authoring and editing numerous books and serving as editor for various journals, he has published more than 90 papers in other countries. Currently serving a term of 2001–2003 as president of the International Federation of the Operational Research Societies (IFORS).
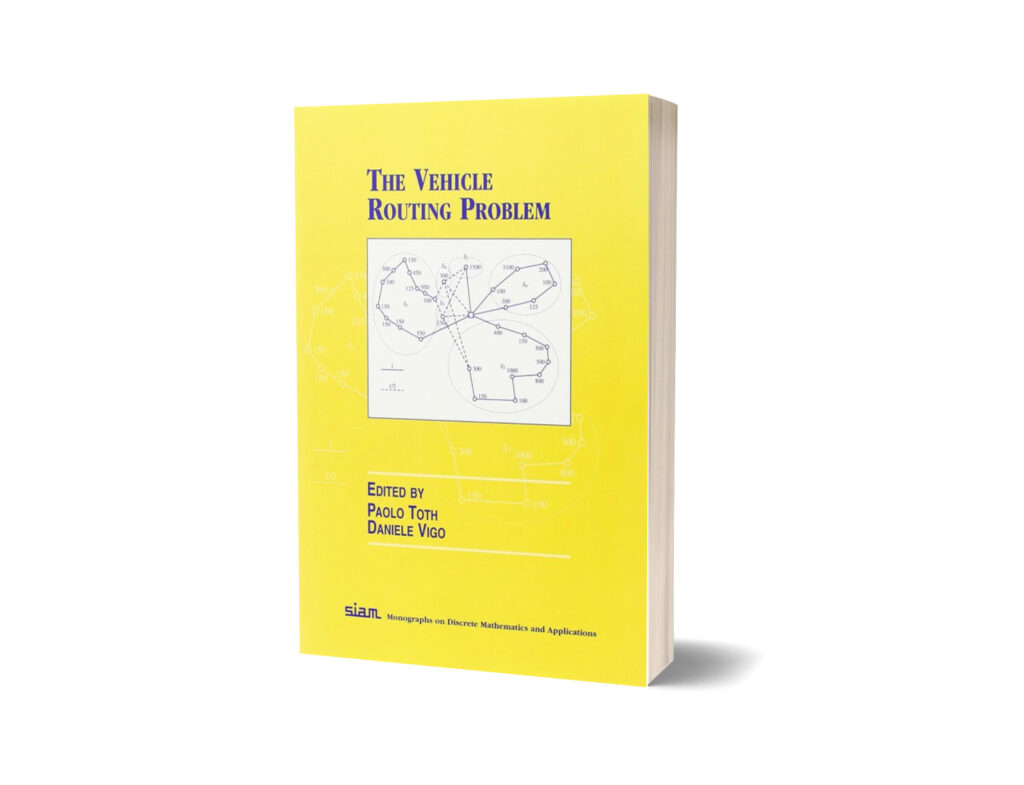
The University of Bologna’s Faculty of Engineering is home to Associate Professor of Operations Research Daniele Vigo. His primary areas of study are in the area of combinatorial optimization, with a focus on algorithms for problems including routing, cutting, packing, and crew management. He is an Associate Editor for the journal Operations Research and has more than 30 publications in other countries.
Table Of Content
1 An Overview of Vehicle Routing Problems R Toth, D. Vigo
1. An Overview of Vehicle Routing Problems R Toth, D. Vigo
1.1 Introduction
1.2 Problem Definition and Basic Notation
1.2.1 Capacitated and Distance-Constrained VRP
1.2.2 VRP with Time Windows
1.2.3 VRP with Backhauls
1.2.4 VRP with Pickup and Delivery
1.3 Basic Models for the VRP
1.3.1 Vehicle Flow Models
1.3.2 Extensions of Vehicle Flow Models
1.3.3 Commodity Flow Model
1.3.4 Set-Partitioning Models
1.4 Test Instances for the CVRP and Other VRPs
Bibliography
I Capacitated Vehicle Routing Problem
2. Branch-and-Bound Algorithms for the Capacitated VRP P. Toth, D. Vigo
2.1 Introduction
2.2 Basic Relaxations
2.2.1 Bounds Based on Assignment and Matching
2.2.2 Bounds Based on Arborescences and Trees
2.2.3 Comparison of the Basic Relaxations
2.3 Better Relaxations
2.3.1 Additive Bounds for ACVRP
2.3.2 Further Lower Bounds for ACVRP
2.3.3 Lagrangian Lower Bounds for SCVRP
2.3.4 Lower Bounds from a Set-Partitioning Formulation
2.3.5 Comparison of the Improved Lower Bounds
2.4 Structure of the Branch-and-Bound Algorithms for CVRP
2.4.1 Branching Schemes and Search Strategies
2.4.2 Reduction, Dominance Rules, and Other Features
2.4.3 Performance of the Branch-and-Bound Algorithms
2.5 Distance-Constrained VRP
Bibliography
3. Branch-and-Cut Algorithms for the Capacitated VRP D. Naddef, G. Rinaldi
3.1 Introduction and Two-Index Flow Model
3.2 Branch-and-Cut
3.3 Polyhedral Studies
3.3.1 Capacity Constraints
3.3.2 Generalized Capacity Constraints
3.3.3 Framed Capacity Constraints
3.3.4 Valid Inequalities from STSP
3.3.5 Valid Inequalities Combining Bin Packing and STSP
3.3.6 Valid Inequalities from the Stable Set Problem
3.4 Separation Procedures
3.4.1 Exact Separation of Capacity Constraints
3.4.2 Heuristics for Capacity and Related Constraints
3.4.3 STSP Constraints
3.5 Branching Strategies
3.6 Computational Results
3.7 Conclusions
Bibliography
4. Branch-and-Cut Algorithms for the Capacitated VRP D. Naddef, G. Rinaldi
4.1 Introduction
4.2 Solving the Linear Programming Relaxation of P
4.3 Set-Covering-Based Solution Methods
4.3.1 Branch-and-Bound Algorithm for Problem CG
4.3.2 Polyhedral Branch-and-Bound Algorithm
4.3.3 Pseudo-Polynomial Lower Bound on cmin
4.3.4 Solving P/> via Dual-Ascent and Branch-and-Bound
4.4 Solving the Set-Covering Integer Program
4.4.1 A Cutting Plane Method
4.4.2 Branch-and-Price
4.4.3 Additional Comments on Computational Approaches
4.5 Computational Results
4.6 Effectiveness of the Set-Covering Formulation
4.6.1 Worst-Case Analysis
4.6.2 Average-Case Analysis
Bibliography
5. Classical Heuristics for the Capacitated VRP G. Laporte, F. Semet
5.1 Introduction
5.2 Constructive Methods
5.2.1 Clarke and Wright Savings Algorithm
5.2.2 Enhancements of the Clarke and Wright Algorithm
5.2.3 Matching-Based Savings Algorithms
5.2.4 Sequential Insertion Heuristics
5.3 Two-Phase Methods
5.3.1 Elementary Clustering Methods
5.3.2 Truncated Branch-and-Bound
5.3.3 Petal Algorithms
5.3.4 Route-First, Cluster-Second Methods
5.4 Improvement Heuristics
5.4.1 Single-Route Improvements
5.4.2 Multiroute Improvements
5.5 Conclusions
Bibliography
6. Metaheuristics for the Capacitated VRP M. Gendreau, G. Laporte, J.-Y. Potvin
6.1 Introduction
6.2 Simulated Annealing
6.2.1 Two Early Simulated Annealing Algorithms
6.2.2 Osman’s Simulated Annealing Algorithms
6.2.3 Van Breedam’s Experiments
6.3 Deterministic Annealing
6.4 Tabu Search
6.4.1 Two Early Tabu Search Algorithms
6.4.2 Osman’s Tabu Search Algorithm
6.4.3 Taburoute
6.4.4 Taillard’s Algorithm
6.4.5 Xu and Kelly’s Algorithm
6.4.6 Rego and Roucairol’s Algorithms
6.4.7 Barbarosoglu and Ozgur’s Algorithm
6.4.8 Adaptive Memory Procedure of Rochat and Taillard
6.4.9 Granular Tabu Search of Toth and Vigo
6.4.10 Computational Comparison
6.5 Genetic Algorithms
6.5.1 Simple Genetic Algorithm
6.5.2 Application to Sequencing Problems
6.5.3 Application to the VRP
6.6 Ant Algorithms
6.7 Neural Networks
Conclusions
Bibliography
II Important Variants of the Vehicle Routing Problem
7. VRP with Time Windows J.-E Cordeau, G. Desaulniers, J. Desrosiers, M. M. Solomon, F. Soumis
7.1 Introduction
7.2 Problem Formulation
7.2.1 Formulation
7.2.2 Network Lower Bound
7.2.3 Linear Programming Lower Bound
7.2.4 Algorithms
7.3 Upper Bounds: Heuristic Approaches
7.3.1 Route Construction
7.3.2 Route Improvement
7.3.3 Composite Heuristics
7.3.4 Metaheuristics
7.3.5 Parallel Implementations
7.3.6 Optimization-Based Heuristics
7.3.7 Asymptotically Optimal Heuristics
7.4 Lower Bounds from Decomposition Approaches
7.4.1 Lagrangian Relaxation
7.4.2 Capacity and Time-Constrained Shortest-Path Problem
7.4.3 Variable Splitting
7.4.4 Column Generation
7.4.5 Set-Partitioning Formulation
7.4.6 Lower Bound
7.4.7 Commodity Aggregation
7.4.8 Hybrid Approach
7.5 Integer Solutions
7.5.1 Binary Decisions on Arc Flow Variables
7.5.2 Integer Decisions on Arc Flow Variables
7.5.3 Binary Decisions on Path Flow Variables
7.5.4 Subtour Elimination and 2-Path Cuts
7.5.5 /c-Path Cuts and Parallelism
7.5.6 Integer Decisions on (Fractional and Integer) Time Variables
7.6 Special Cases
7.6.1 Multiple TSP with Time Windows
7.6.2 VRP with Backhauls and Time Windows
7.7 Extensions
7.7.1 Heterogeneous Fleet, Multiple-Depot, and Initial Conditions
7.7.2 Fleet Size
7.7.3 Multiple Time Windows
7.7.4 Soft Time Windows
7.7.5 Time-and Load-Dependent Costs
7.7.6 Driver Considerations
7.8 Computational Results for VRPTW
7.9 Conclusions
Bibliography
8. VRP with Backhauls P. Toth, D. Vigo
8.1 Introduction
8.1.1 Benchmark Instances
8.2 Integer Linear Programming Models
8.2.1 Formulation of Toth and Vigo
8.2.2 Formulation of Mingozzi, Giorgi, and Baldacci
8.3 Relaxations
8.3.1 Relaxation Obtained by Dropping the CCCs
8.3.2 Relaxation Based on Projection
8.3.3 Lagrangian Relaxation
8.3.4 Overall Additive Lower Bound
8.3.5 Relaxation Based on the Set-Partitioning Model
8.4 Exact Algorithms .
8.4.1 Algorithm of Toth and Vigo
8.4.2 Algorithm of Mingozzi, Giorgi, and Baldacci
8.4.3 Computational Results for the Exact Algorithms
8.5 Heuristic Algorithms
8.5.1 Algorithm of Deif and Bodin
8.5.2 Algorithms of Goetschalckx and Jacobs-Blecha
8.5.3 Algorithm of Toth and Vigo
8.5.4 Computational Results for the Heuristics
Bibliography
9. VRP with Pickup and Delivery G. Desaulniers, J. Desrosiers, A. Erdmann, M. M. Solomon, F. Soumis
9.1 Introduction
9.2 Mathematical Formulation
9.2.1 Construction of the Networks
9.2.2 Formulation
9.2.3 Service Quality
9.2.4 Reduction of the Network Size
9.3 Heuristics
9.3.1 Construction and Improvement
9.3.2 Clustering Algorithms
9.3.3 Metaheuristics
9.3.4 Neural Network Heuristics
9.3.5 Theoretical Analysis of Algorithms
9.4 Optimization-Based Approaches
9.4.1 Single Vehicle Cases
9.4.2 Multiple Vehicle Cases
9.5 Applications
9.6 Computational Results
9.7 Conclusions
Bibliography
III Applications and Case Studies
10. Routing Vehicles in the Real World: Applications in the Solid Waste, Beverage, Food, Dairy, and Newspaper Industries B. L. Golden, A. A. Assad, E. A. Wasil
10.1 Introduction
10.2 Computerized Vehicle Routing in the Solid Waste Industry
10.2.1 History
10.2.2 Background
10.2.3 Commercial Collection
10.2.4 Residential Collection
10.2.5 Case Studies
10.2.6 Roll-on-Roll-off
10.2.7 Further Remarks
10.3 Vehicle Routing in the Beverage, Food, and Dairy Industries
10.3.1 Introduction
10.3.2 Beverage Industry
10.3.3 Food Industry
10.3.4 Dairy Industry
10.4 Distribution and Routing in the Newspaper Industry
10.4.1 Industry Background
10.4.2 Newspaper Distribution Problem
10.4.3 Vehicle Routing Algorithms for NDP
10.4.4 Three Case Studies
10.4.5 Further Remarks
10.5 Conclusions
Bibliography
11. Capacitated Arc Routing Problem with Vehicle-Site Dependencies: The Philadelphia Experience J. Sniezek, L. Bodin, L. Levy, M. Bal
11.1 Introduction
11.2 Networks, Assumptions, and Goals of the CARP-VSD
11.2.1 Travel Network
11.2.2 Service Network
11.2.3 Vehicle Classes
11.2.4 Travel Network and Service Network for a Vehicle Class
11.2.5 Vehicle Preference List
11.2.6 Other Assumptions
11.2.7 Goals and Constraints of the CARP-VSD
11.3 Vehicle Decomposition Algorithm (VDA)
11.3.1 Step A. Create and Verify Vehicle Class Networks
11.3.2 Step B. Estimate Total Work and Determine Initial Fleet Mix
11.3.3 Step C. Partition the Service Network
11.3.4 Step D. Determine Travel Path and Balance the Partitions
11.3.5 Step E. Revise Estimate of Total Work and Adjust Fleet Mix
11.4 Implementation of the VDA in Philadelphia
11.5 Enhancements and Extensions
Bibliography
12. Inventory Routing in Practice Ann M. Campbell, Lloyd W. Clarke, Martin W.R Savelsbergh
12.1 Introduction
12.2 Problem Definition
12.3 Literature Review
12.4 Solution Approach
12.4.1 Phase I: Integer Programming Model
12.4.2 Phase I: Solving the Integer Programming Model
12.4.3 Phase II: Scheduling
12.5 Computational Experience
12.5.1 Instances
12.5.2 Solution Quality
12.5.3 Alternate Heuristic
12.5.4 Computational Experiments
12.6 Conclusion
Bibliography
13. Routing Under Uncertainty: An Application in the Scheduling of Field Service Engineers E. Hadjiconstantinou, D. Roberts
13.1 Introduction
13.2 VRPSST with Variable Costs of Recourse
13.3 Literature Review
13.3.1 VRPST
13.3.2 VRPSD
13.4 Stochastic Integer VRPSST Formulation
13.4.1 First-Stage Problem
13.4.2 Second-Stage Problem
13.5 Paired Tree Search Algorithm (PTSA)
13.5.1 Linked Trees
13.5.2 Lower Bounds
13.5.3 Computational Implementation
13.6 Applied Maintenance Scheduling Problem
13.6.1 Maintenance Scheduling System in Practice
13.6.2 Stochastic Problem Setting
13.7 Modeling the Applied Problem as a VRPSST
13.8 Model Input
13.8.1 Job Locations and the Road Network
13.8.2 Service Times
13.9 Model Output: Computational Considerations
13.9.1 Framework for the Analysis of Results
13.9.2 Reoptimization
13.10 Example Scenario
13.11 Overall Computational Results
13.12 Conclusion
Bibliography
14. Evolution of Microcomputer-Based Vehicle Routing Software: Case Studies in the United States E. K. Bake
14.1 Introduction
14.2 Definition of the VRP
14.2.1 Customer Specification
14.2.2 Product Specification
14.2.3 Vehicle Specification
14.3 Algorithms
14.4 Future Trends in Vehicle Routing Software
14.5 Summary and Conclusions
Bibliography
Index